
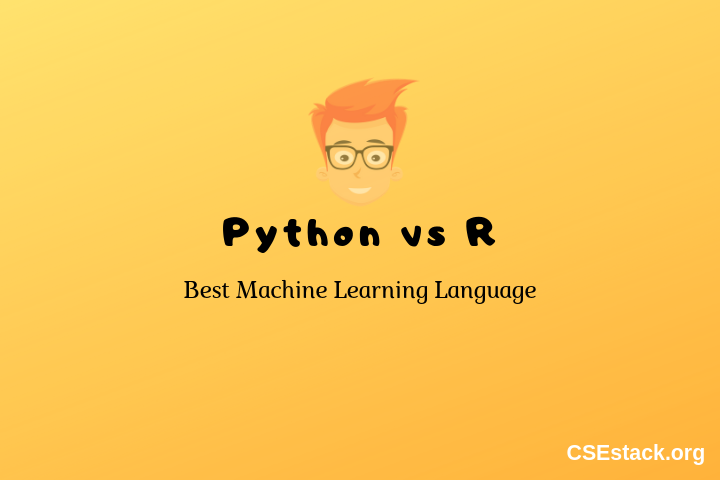

- Ide for r and python how to#
- Ide for r and python archive#
- Ide for r and python software#
- Ide for r and python code#
Ide for r and python code#
A number of times these libraries are written in a language like C while the user writes the code in R, making R more efficient and faster than several other languages.ĪnalytixLabs is the premier Data Analytics Institute that specializes in training individuals as well as corporates to gain industry-relevant knowledge of Data Science and its related aspects.
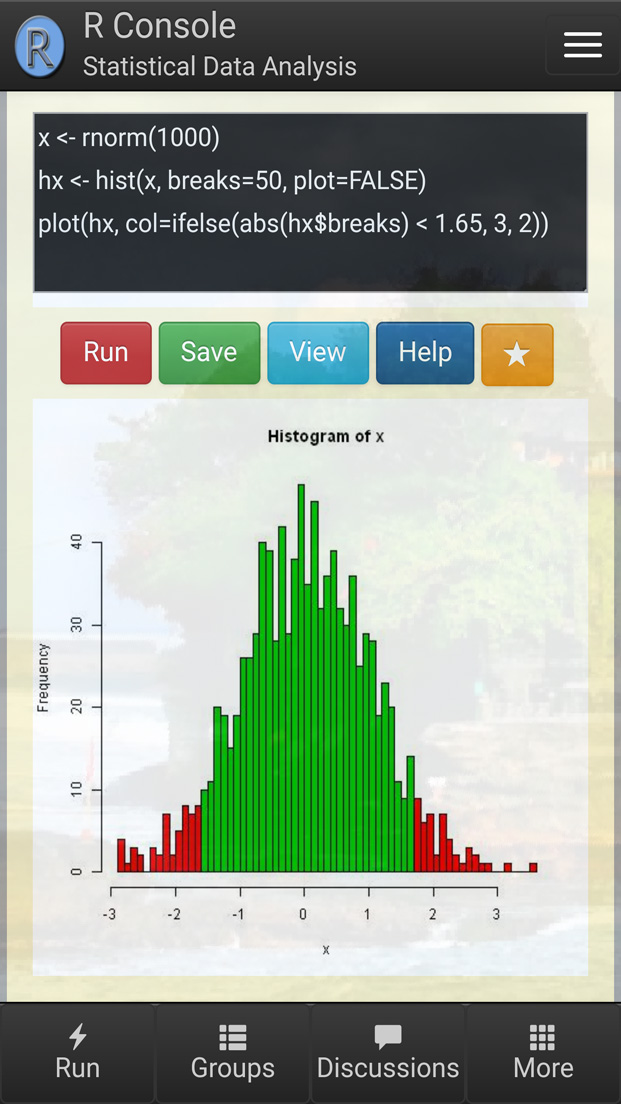
Ide for r and python archive#
These libraries can be extended by the users by creating their own functions to perform operations in R and making them publicly available through CRAN (Comprehensive R Archive Network) which is a family of connected servers that provide R with unprecedented functionalities. R has a very strong concept of the environment within which a number of functions lie that are accessible through libraries/modules. Users can even write code in C to manipulate the R’s objects. In order to perform computationally heavy operations, low-level languages such as C and C++ can be linked with R.
Ide for r and python software#
Being free software it works under the terms of the GNU General Public License in source code form which is provided by the Free Software Foundation. Created as a part of the GNU project which uses the GNU operating system and its packages to create free software, it has since been maintained by the R foundation (which is a non-profit organization).
Ide for r and python how to#
The aim of this article is to provide the readers with a decent knowledge of what is r programming language, its basics, usage and answer how to learn r programming language and also some information about how it is different from its prime competitors – python.Ĭreated by Ross Ihaka and Robert Gentleman in 1995 (and released in 1997), R is a low-level language created to implement the S language with ease. This consequently has led to a number of tools taking a centre stage when it comes to the common and important tools used for performing the various operations that relate to data. This has led to a huge surge in the demand for business analysts, data analysts and data scientists. The pace at which data is being generated and the quality of data have increased exponentially hiding a great deal of information in them. Julia’s tools did not seem as developed for these purposes.Almost all organisations have a data science department or are involved in some data related operations. I tried using Julia to complete some of the basic tasks I now do in R. The community of R developers, particularly the rockstar data scientist Hadley Wickham, have developed terrific tools, with thorough documentation, for doing simple data analysis tasks. I am an R user, and most of the statistics work I do is on relatively small datasets, and involves simple calculations. One, if processing speed isn’t important to you, Julia is probably inferior to whatever product you are using-at least for now. So, why shouldn’t every data scientist learn Julia? There are a couple of reasons. These developers now are only a small part of Julia’s progression, with over 700 volunteers contributing to the 1.0 version of the software. Julia’s other two creators are Jeff Bezanson and Stefan Karpinski (the name of the language came from an old project’s of Bezanson’s). Most of the key developments in Julia now come from MIT’s Julia Lab, which is led by fellow Julia creator and MIT mathematics professor Alan Edelman. The New York Federal Reserve and the investment firm Blackrock are among its customers. Shah nows runs Julia Computing, a consultancy that helps other companies implement Julia. It hopes that Julia will overtake Python and R as the central language for data science, and particularly for machine learning. Julia is already widely used, with over 2 million people having downloaded it, but the community of users has bigger ambitions. Julia is faster than Python and R because it is specifically designed to quickly implement the basic mathematics that underlies most data science, like matrix expressions and linear algebra. Then, when they were happy with the algorithm, they would rewrite the program in C++ or Java to get fast computer processing performance. Data scientists would first use a tool like Python or R to develop an algorithm, because it was easy to explore the data and make charts in those languages. Shah says that the key inspiration for developing Julia was seeing how many people had to write the same program twice. “In Julia, we created a language that was simultaneously fast and easy.” “If you are a mathematician, scientist, or engineer, you have historically had the choice to pick a language that was fast, like C++ or Java, or a language was easy to learn, like Matlab, R, or Python,” says Viral Shah, one of the creators of Julia.
